Your Guide to Understanding Statistical Significance
February 5, 2023
·Ahmad Jadallah
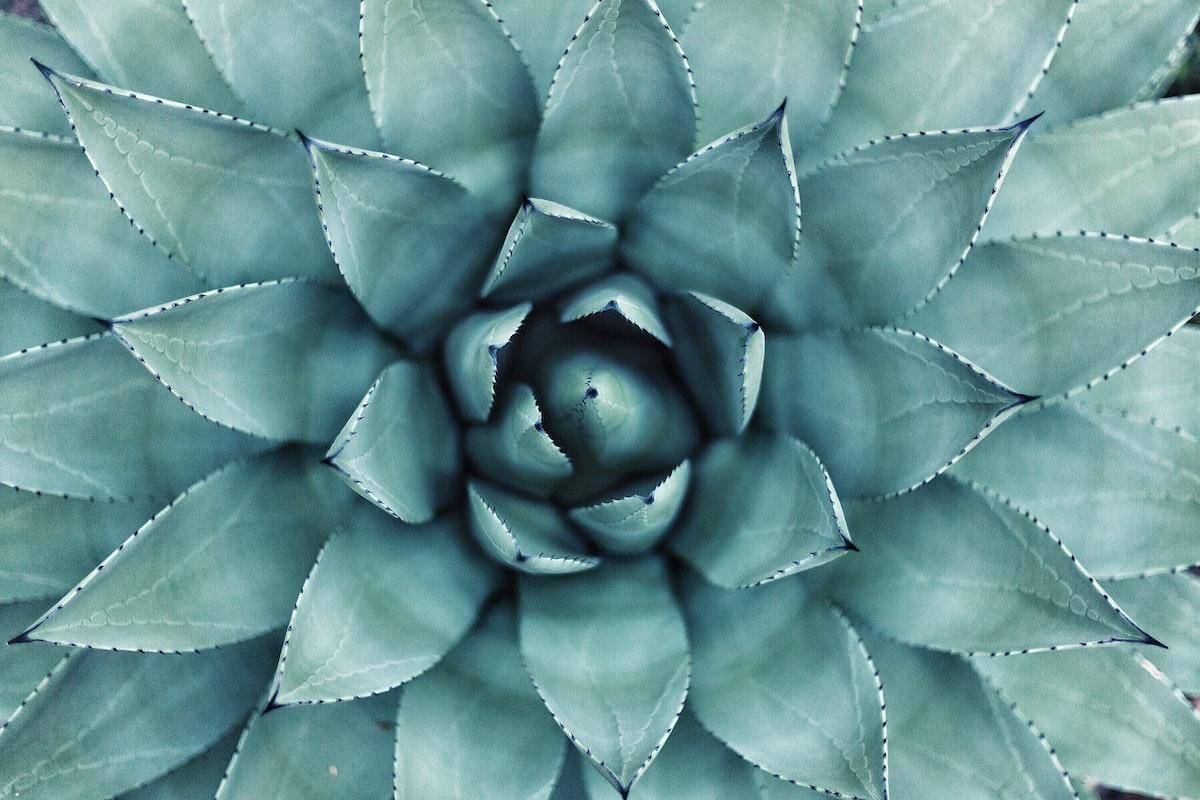
What does it mean to provide evidence using data?
Here is where statistical significance comes in hand.
Imagine yourself as the dean of a prestigious institution, you get an alarming report indicating that your students average 6.80 hours of sleep each night, compared to the national average of 7.02 hours. The student body president is concerned about the kids’ health and cites this report as evidence that homework should be lowered. In contrast, the university president dismissed the report as nonsense: “Back in my day, we considered ourselves fortunate if we got four hours of sleep a night.” You must determine whether this is a serious matter. You are well-versed in statistics and see an opportunity to put your knowledge to work!
Statistical significance is one of those concepts that we frequently hear but rarely comprehend. When someone asserts that data validate their thesis, we nod and accept it, assuming that statisticians have performed intricate calculations that have produced an unquestionable outcome. In reality, statistical significance is not a complex phenomenon that requires years of study to acquire; rather, it is a simple concept that everyone can and should grasp. As is the case with the majority of technical topics, statistical significance is based on a few elementary principles: hypothesis testing, the normal distribution, and p value. As we work towards resolving the aforementioned problem, we will touch briefly on each of these principles in this post.
Testing hypotheses, a method for assessing a theory using facts, is the first concept we must address. The “hypothesis” refers to the researcher’s pre-study belief of the issue. This first notion is referred to as the alternative hypothesis, whereas its opponent is referred to as the null hypothesis. In our case, they include:
- Alternative Hypothesis: The average quantity of sleep that students at our university get is less than the national average for college students.
- Null Hypothesis: The average number of hours slept by students at our institution is not less than the national average for college students.
Observe how cautious we must be with the language: we are searching for a very particular impact, which must be specified in the hypotheses so that we cannot later argue that we were testing anything different! (This is an example of a one-sided hypothesis test because we are only interested in one direction of change.) Hypothesis testing are one of the pillars of statistics and are used to evaluate the outcomes of the vast majority of investigations. These studies might range from a clinical trial to test the efficacy of a medicine to an observational study to evaluate an exercise regimen. All studies are focused with drawing comparisons between two groups or between one group and the total population. In the medical example, we may compare the average time to recover between groups using two different medications, but in our situation as deans, we wish to compare sleep between our students and those of the entire nation.
The testing portion of hypothesis tests helps us to identify whether theory, the null or alternative, is supported by the data more strongly. There are several hypothesis tests; we will utilize the z-test. However, before we can evaluate our data, we must discuss two other essential concepts.
The second component of statistical significance is the normal distribution, commonly known as the Gaussian distribution or bell curve. The normal distribution is used to depict the distribution of data from a process and is characterized by the mean, denoted by the Greek letter (mu), and the standard deviation, denoted by the letter (sigma) (sigma). The mean indicates the location of the data’s center, while the standard deviation represents the data’s dispersion.
The normal distribution is used when evaluating data points in terms of the standard deviation. Based on the number of standard deviations a data point deviates from the mean, we may evaluate its degree of anomaly. The normal distribution has the following advantageous characteristics:
- 68% of the data falls within one standard deviation of the mean.
- 95% of the data fall within two standard deviations of the mean.
- 99.7 percent of the data falls within three standard deviations of the mean.
If a statistic has a normal distribution, each point may be described in terms of standard deviations from the mean. For instance, the mean female height in the United States is 65 inches with a standard variation of 4 inches. If a new acquaintance is 73 inches tall, she is two standard deviations above the mean and among the tallest 2.5% of females. (2.5% of females will be shorter than average — 2(sigma) (57 in) and 2.5% will be taller than average + 2(sigma) (59 in)).
Instead of stating that our data is two standard deviations from the mean, we evaluate it using a z-score, which simply reflects the number of standard deviations a point is from the mean. Subtracting the mean of the distribution from the data point and dividing by the standard deviation yields a z-score. In the height example, you can verify that our friend’s z-score would be 2. The resulting distribution is the standard normal with a mean of 0 and a standard deviation of 1, as seen in the figure below.
Every time we do a hypothesis test, we must assume a distribution for the test statistic, which in our case is the mean (average) number of hours our kids sleep. The normal curve is used as an estimate for the distribution of the test statistic when conducting a z-test. According to the central limit theorem, when more averages are extracted from a data distribution, the averages converge toward a normal distribution. Nonetheless, this is always an approximation, as real-world data never fully adhere to a normal distribution. The assumption of a normal distribution enables us to assess the significance of the results of a research. The higher or lower the z-score, the less probable it is that a result occurred by chance and the more likely it is that the result is significant. To quantify the significance of the results, we employ one additional notion.
The final fundamental concept is that of p-values. A p-value is the likelihood of observing outcomes that are at least as severe as those observed under the null hypothesis. This may appear quite complicated, so let’s examine an illustration.
Suppose we are comparing the average IQ of Florida and Washington. The null hypothesis is that the average IQs in Washington are not greater than those in Florida. Using a p-value of 0.346, we determine that IQs in Washington are higher by 2.2 points. In a universe in which the null hypothesis — that average IQs in Washington are not higher than average IQs in Florida — holds true, there is a 34.6% likelihood that we would test IQs that are at least 2.2 points higher in Washington. Therefore, if IQs in Washington are not genuinely higher, we would still find them to be at least 2.2 points higher around one-third of the time owing to random noise. The lower the p-value, the more significant the finding, as it is less likely to be produced by noise.
Whether or not a result may be deemed statistically significant relies on the significance p-value (also known as alpha) established before to the experiment. The results are statistically significant if the observed p-value is smaller than alpha. If we waited until after the experiment to determine alpha, we could just choose a value that ensures our results are significant regardless of what the data indicates!
The choice of alpha depends on the context and the topic of research, but the most frequently employed number is 0.05, which corresponds to a 5 percent possibility that the results are random. In my laboratory, numbers between 0.1 and 0.001 are utilized often. The researchers who found the Higgs Boson particle, as an extreme example, chose a p-value of 0.0000003, or a 1 in 3.5 million probability that the finding was due to noise. (Statisticians are hesitant to acknowledge that a p-value of 0.05 is arbitrary. R.A. Fischer, the inventor of modern statistics, arbitrarily chose a p-value of 0.05, and it stayed)!
To convert a normal distribution z-score to a p-value, we can use a table or statistical program such as R. The result will indicate the likelihood of a z-score less than the computed number. For instance, given a z-score of 2, the p-value is 0.977, indicating that there is only a 2.3% chance of randomly seeing a z-score greater than 2.
In summary, we have thus far discussed three concepts:
- Hypothesis Testing: A method for evaluating an idea
- Normal Distribution: An approximation of the data used to test a hypothesis.
- p-value: The likelihood that a result at least as dramatic as the observed one would have occurred if the null hypothesis were true.
Now, let’s put the parts of our example together. Here are several essentials:
According to the National Sleep Foundation, students throughout the country sleep 7.02 hours each night on average.
In a survey of 202 university students, the average number of hours slept per night was 6.90, with a standard deviation of 0.80 hours.
Our alternate hypothesis is that students at our institution sleep less than the national average for college students.
We will choose an alpha value of 0.05, which indicates that the results are statistically significant if the p-value is less than 0.05.
First, we must transform our measurement into a z-score, which indicates the number of standard deviations from the mean. This is accomplished by subtracting the population mean (the national average) from the measured value and dividing by the standard deviation over the square root of the sample size. (As the number of samples rises, the variance and standard deviation drop. This is taken into consideration by dividing the standard deviation by the square root of the sample size.)
The z-score is referred to as the test statistic. Once we have a test statistic, we may compute the p-value using a table or a programming language such as R. I utilize code to demonstrate how simple it is to implement our approach with free software. (# denotes comments; bold indicates output)
# Calculate the results
z_score = (6.90 - 7.02) / (0.84 / sqrt(202))
p_value = pnorm(z_score)# Print our results
sprintf('The p-value is %0:5f for a z-score of %0.5f.', p_value, z_score)"The p-value is 0.02116 for a z-score of -2.03038."
Based on the p-value of 0.02116, the null hypothesis may be rejected. (Statisticians prefer that we reject the null hypothesis instead than accepting the alternative.) At a significance level of 0.05, there is statistically significant evidence that our pupils sleep less on average than college students in the United States. The p-value indicates that there is a 2.12% probability that our results are due to random noise. In this presidential debate, the student was correct.
Before banning all schoolwork, we must be cautious not to place too much emphasis on this result. Notice that our p-value, 0.02116, would not be statistically significant if we had used a cutoff of 0.01. Those who wish to refute the findings of our study can easily modify the p-value. In addition to the conclusion, we should consider the p-value and sample size whenever we review a study. With a sample size of 202, our study may have statistical significance, but this does not imply that it is practically significant. In addition, because this was an observational research, there is just evidence for association and not causality. We demonstrated that there is a link between students at our school and decreased average sleep, but not that attending our school causes sleep loss. There may be other variables influencing sleep, and only a randomized controlled research can confirm causality.
As is the case with the majority of technical notions, statistical significance is comprised of several simple principles. The most of the difficulty is in acquiring the vocabulary! After assembling the necessary components, you may begin using these statistical principles. As you master the fundamentals of statistics, you will be better equipped to examine studies and the news with a healthy degree of skepticism. You are able to see what the data truly says, as opposed to what someone claims it implies. The most effective strategy against dishonest politicians and businesses is an informed and suspicious populace!
Related Posts
- Bioinformatics
- Genomic
Unlocking the Potential of Genomic Medicine with Bionl
Genomic medicine, a branch of medical science that uses genomic information to guide patient care, is heralding a new era in healthcare. By analyzing an individual’s genetic makeup, healthcare professionals can make more informed decisions regarding the diagnosis, treatment, and prevention of diseases. In this post, we will delve into the fascinating world of genomic medicine and explore how Bionl is playing a pivotal role in advancing this field.
Jul 2023
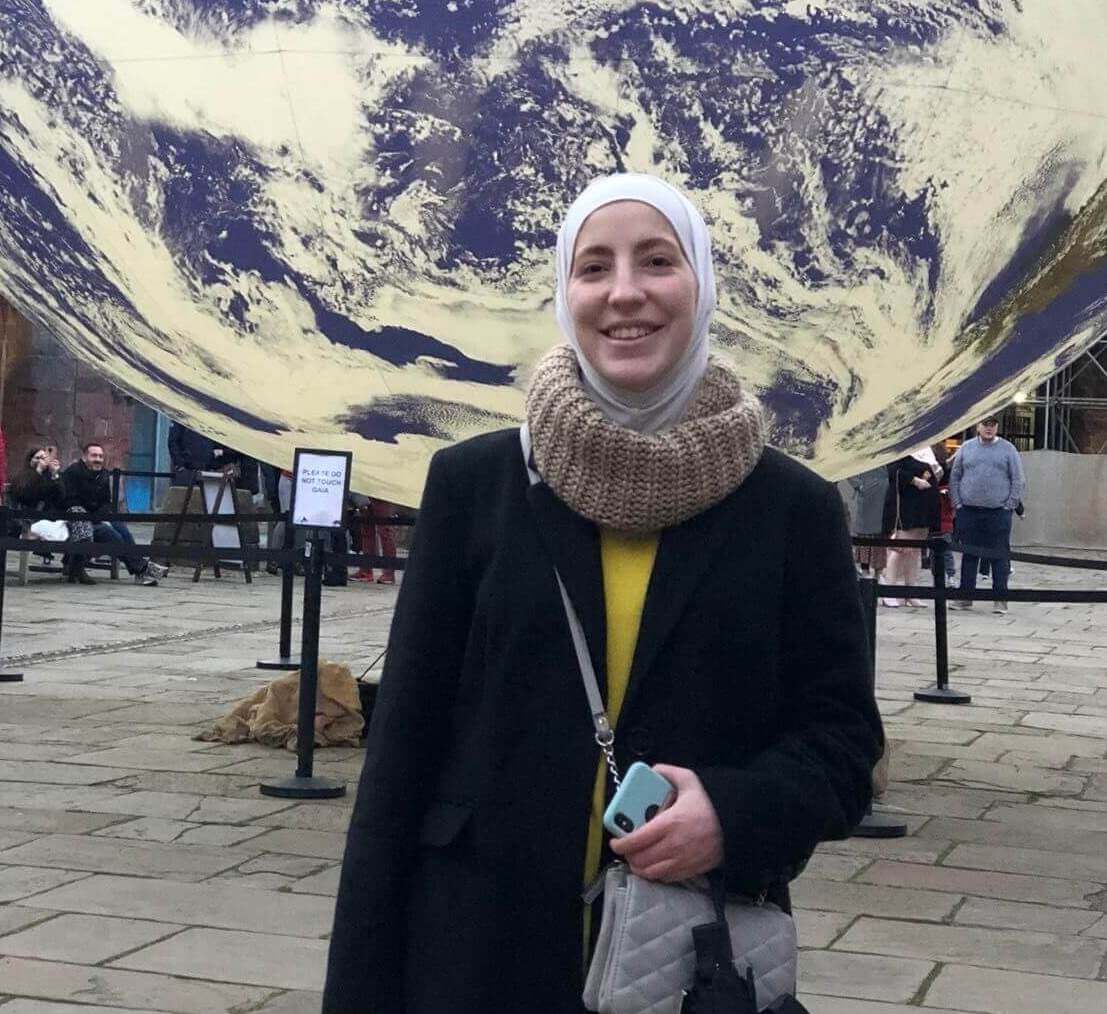
Tasnim Nour
- Bioinformatics
- Research
Bioinformatics Research: Illuminating the Path of Evolution
Bioinformatics, a field at the intersection of computer science and biology, is growing at a remarkable pace. It empowers researchers to collect and analyze vast datasets related to genes, proteins, and various biological molecules, revolutionizing our understanding of life's intricate processes.
Nov 2023
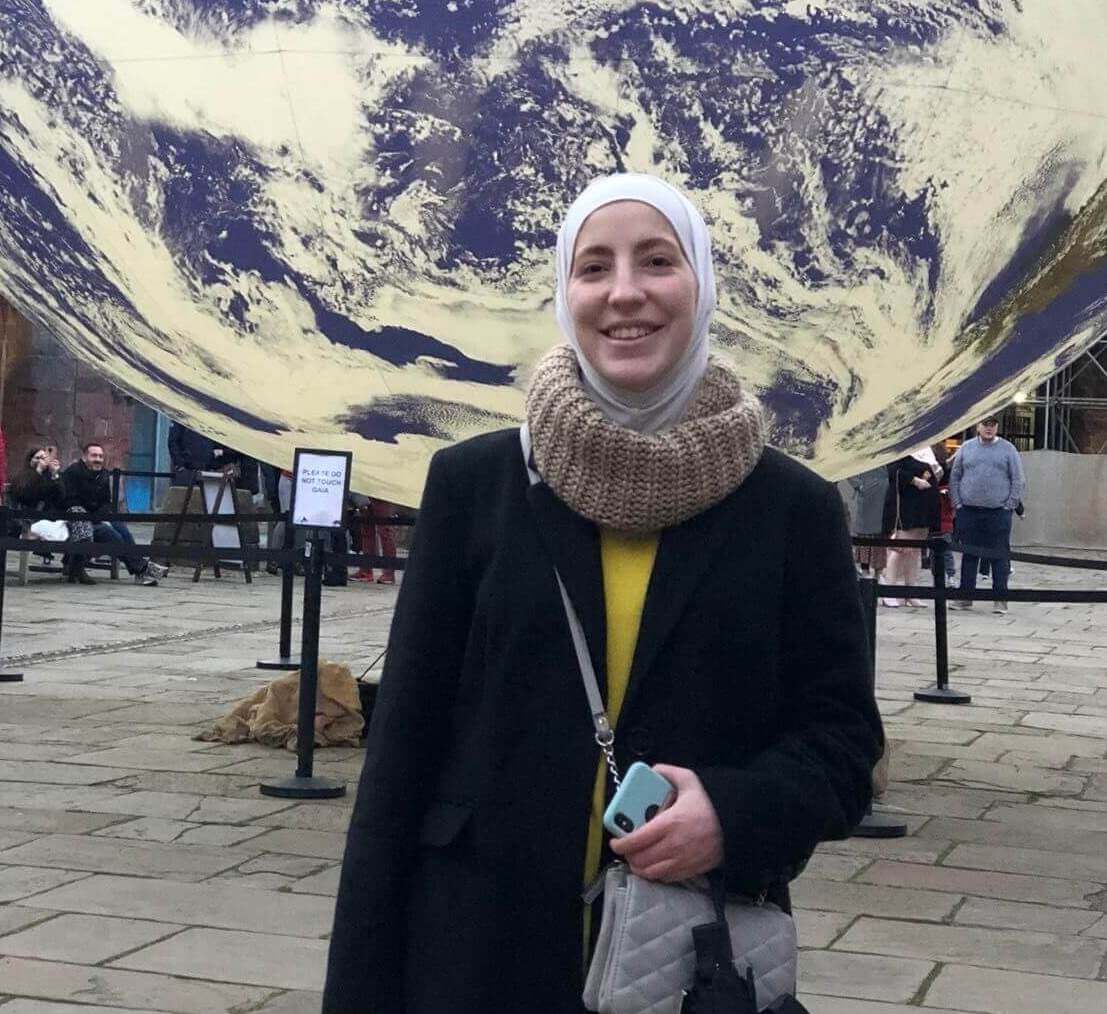